How would you react if ChatGPT revealed your phone number and home address?
Among the data on the web that the language model behind ChatGPT trains on, some information is personal and is not intended to be revealed. However, this risk is very real, as a team of researchers demonstrated by pushing ChatGPT to reveal a large amount of personal data from a simple request .
To guarantee the confidentiality of the data used to train artificial intelligence systems, one option is to use “synthetic data”: artificially generated fictitious data that retains statistical properties of the real data set that it seeks to imitate. and replace.
With synthetic data, we can train a classification system or a conversational agent like ChatGPT, test software, or share data without concern for confidentiality: synthetic data reproduce, for example, data from the national health data system .
Some of the companies present in the synthetic data generation market, as well as part of the academic literature, even argue that this is truly anonymous data . This term is strong, because it implies that we cannot go back to the real data – and therefore, to your social security or telephone number.
In reality, the data synthesis has weaknesses and the guarantees put forward are still the subject of studies .
Why so much enthusiasm for synthetic data?
Data synthesis would make it possible to publish data that is representative of the original real data but not identifiable.
For example, population census data can be extremely useful for statistical purposes… but they gather information on individuals which allows their re-identification: their publication as is is therefore not permitted by the GDPR (Regulation general data protection) .
In the case of personal data or data subject to intellectual property, this process would also make it possible to overcome the regulatory framework which often limits their publication or use.
It would also make it possible to carry out experiments that would have required costly data collection, for example to train autonomous cars to avoid collisions .
Finally, synthetic data does not require data cleaning. This asset is particularly important for training AI models, where the quality of data annotation impacts model performance.
For these reasons, by the end of 2022, the global synthetic data generation market had already generated $163.8 million and is expected to grow by 35% from 2023 to 2030 . Adoption could be rapid and massive, and according to some studies represent up to 60% of the data used for training AI systems in 2024 .
Privacy is one of the goals of generating synthetic data, but it is not the only one. Players in the field also intend to take advantage of the exhaustiveness of synthetic data – which can be generated in almost unlimited quantities and reproduce all the simulations envisaged, but also make it possible to have data on particularly difficult cases with real data (such as the detection of weapons on an image, or a simulation of road traffic with very specific conditions).
How do we generate synthetic data?
Let’s imagine that we want to generate synthetic data such as the age and salary of a population. We first model the relationship between these two variables, then we exploit this relationship to artificially create data satisfying the statistical properties of the original data.
If data synthesis was initially based on statistical methods, the techniques are today more sophisticated, in order to synthesize tabular or temporal data – or even, thanks to generative AI, data such as text, images, voice and videos .
In fact, the techniques used to synthesize data for confidentiality purposes are very similar to those used by generative AIs such as ChatGPT for text, or StableDiffusion for images. On the other hand, an additional constraint linked to the reproduction of the statistical distribution of the source data is imposed on the tools in order to ensure confidentiality.
For example, generative adversarial networks (or GANs ) can be used to create deepfakes .
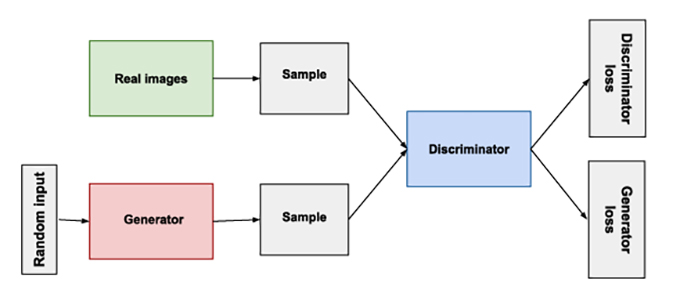
Schematic operation of a generative adversarial network, or GAN: the basic idea of GANs is to oppose two distinct neural networks, the generator and the discriminator. As the generator creates new data, the discriminator evaluates the quality of that data. The two networks train in a loop, thus improving their respective performances. The process ends when the discriminator is no longer able to discern real data from that coming from the generator. Vincent Barra
For their part, variational autoencoders (or VAEs) compress the original data into a lower dimensional space and attempt to model the distribution of this data in this space. Random points are then drawn from this distribution and decompressed to create new data faithful to the original data.
There are other generation methods . The choice of method depends on the source data to be imitated and its complexity.
Real data vs. synthetic data: finding the differences
The modeling of the original data, on which the synthesis process is based, may be imperfect, erroneous or incomplete. In this case, the summary data will only partially reproduce the original information: we speak of a “loss of utility”.
Beyond a loss in performance, a poorly trained or biased data generator can also have an impact on minority groups, underrepresented in the training dataset, and therefore less well assimilated by the model.
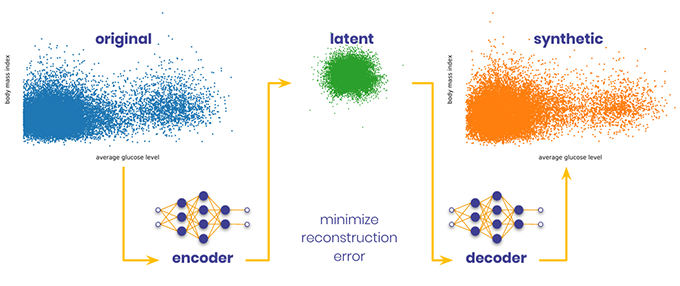
Example of generating synthetic data that is faithful to the training data on the left, and which does not faithfully reproduce the training data on the right. The data here is represented by “principal components” analysis, a type of matrix statistical analysis that is very common in the data world.
The loss of usefulness is a risk that is all the more worrying because only the organization at the source of the synthesis is able to estimate it, leaving data users in the illusion that the data corresponds to their expectations.
Synthetic data vs. anonymous data: what guarantee in terms of confidentiality?
When sharing personal data is not permitted, personal data must be anonymized before being shared . However, anonymization is often technically difficult, or even impossible for certain datasets.
Synthetic data is then placed as a replacement for anonymized data. However, as with anonymized data, zero risk does not exist .
Although all of the source data is never revealed, the summary data, and sometimes the generation model used, can be made accessible and thus constitute new attack possibilities.
To quantify the risks associated with the use of synthetic data, privacy properties are evaluated in different ways:
- Ability to link synthetic data to original data
- Attribute Disclosure : When access to synthetic data allows an attacker to infer new private information about a specific individual, for example the value of a particular attribute like race, age, income, etc.
- Membership inference : for example, if an adversary discovers that an individual’s data was used to train a model that predicts the risk of cancer recurrence, they can derive information about that individual’s health. individual.
Non-zero risks
It is important to understand that in most cases, the risk of information leakage is not binary: confidentiality is neither total nor zero.
Risk is assessed through probability distributions based on the assumptions, data and threats considered. Some studies have shown that synthetic data offers little additional protection compared to anonymization techniques. Furthermore, the trade-off between privacy of the original data and utility of the synthetic data is difficult to predict.
Certain technical measures make it possible to reinforce confidentiality and reduce the risks of re-identification. Differential confidentiality in particular is a promising solution, still under study in order to provide sufficient guarantees in terms of data usefulness, computational cost and absence of bias .
It should still be noted that although the risks associated with the use of synthetic data are not zero, their use can prove advantageous for certain use scenarios. For example, we can calibrate the generation so that it only retains certain properties of the source data to limit risks.
As with any protection solution to be implemented, it is always necessary to carry out a risk analysis to objectivize your choices . And of course, the generation of synthetic data also raises ethical issues when the purpose of data generation is to construct false information .
Author Bios: Antoine Boutet is a Lecturer, Privacy, AI, at the CITI laboratory, Inria at INSA Lyon – University of Lyon and Alexis Leautier is an Expert Engineer at the Digital Innovation Laboratory at CNIL