As the holidays approach, many are wondering if they’ll actually get that expensive gift they asked for this year. Community college presidents are hoping for a big gift too–one that will help them understand whether they are achieving their college access and success goals.
Earlier this fall, The League for Innovation in the Community College, an international organization of 800 member colleges dedicated to innovation, experimentation, and institutional transformation, identified seven key trends that will challenge their institutions in the years ahead. The League sent a survey to over 1,000 community college presidents and asked them to rate the extent to which priorities around access and completion would take place at their institutions.
Results from the 2014 survey were highlighted in the report, League for Innovation Trends Report. Different from previous years (the first survey was conducted in 1997) is the major move from a focus on accountability analytics—conversations and innovations focused on getting data to administrators, legislators, and trustees—to action analytics, where the focus is on getting real-time and predictive data into the hands of teachers, advisors, and students on the front lines of learning.
Predictive analytics is the use of modeling to pinpoint variables that can help predict the aspects of the student experience that contribute to academic success. The value of predictive analytics is in building on an analysis of those variables to design interventions that improve student progress.
An example would be instead of only having real-time data to power early warning systems that signals when a student is having difficulty in a course, colleges create predictive models (from historical data the institution or a group of institutions already collects) that suggested which students might be at risk before they had a chance to steer off track and could further guide strategic and proactive outreach to improve student completion. Once a college has a statistical model, they could use it again and again to predict future behavior, or revise it as additional data became available.
Sinclair Community College’s Pathways to Completion (PTC) initiative uses predictive analytics to identify first-time-in-college, at-risk students and assigns them a Student Success Coach before they register for courses. Students are supported through case management software, academic advising tools, and early alert systems if they start going off track academically.
The program is already showing early success. For example, the year-to-year retention rate of active participants in the PTC program is 40 percentage points higher than similar students who are not in the PTC program, and the average year-to-year retention of PTC minority students is over 50 percentage points higher than non PTC students. PTC students are also five time more likely to graduate within 6 years.
Furthermore, predictive analytics could be used to help improve outcomes for traditionally underserved students, something community college leaders sorely want. Almost half (46.6 percent) of community college presidents responded yes to expanding the use of data to better understand and serve different student types, and a third responded yes enthusiastically.
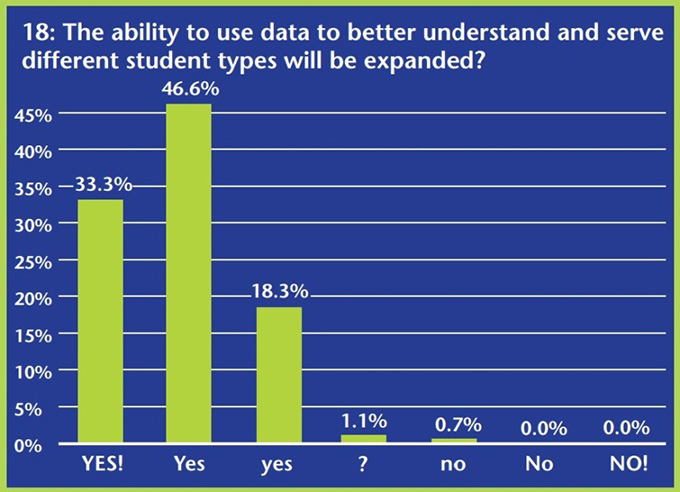
Source: de los Santos, G. E., & Milliron, M. (2015). League for Innovation Trends Report. Chandler, AZ: League for Innovation in the Community College.
Trends Report. Chandler, AZ: League for Innovation in the Community College.
Action and predictive analytics won’t come by wishful thinking however. At minimum, community colleges will need resources (both people and financial), to figure out what questions they hope to answer with analytics, and how to safeguard against inadvertently using the new tools and analytic capabilities to harm the very students they have in mind to help. Additionally, how institutions will build out their data infrastructure to support such analysis and tools should also be considered.
Action and predictive analytics are the new shiny toy in higher education, and that’s a good thing because as some amount of postsecondary education becomes increasingly necessary for economic security, new approaches to ensure students succeed should be welcomed.